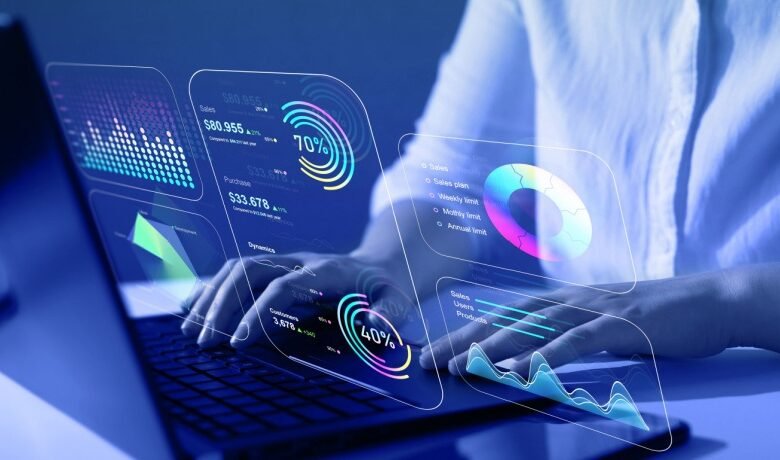
Which Data Analysis Processes Are Necessary For Effective eLearning?
The advancement of eLearning in recent years is undeniable. With the evolution of technology, Instructional Designers are able to create effective learning experiences that allow for flexible and user-directed learning. One way they’ve been able to achieve this is by leveraging the power of data to gain valuable insights. In this article, we discuss the significance of data analysis for eLearning, followed by the most common data analysis processes and types of analytics you can use to ensure the effectiveness of your eLearning course.
How Does Data Analysis Support eLearning Success?
Data analysis is crucial for the success of any eLearning program, as it provides a deep understanding of learner engagement, performance, and preferences. It discovers patterns, trends, and interactions in the large volume of data generated from LMSs and other eLearning platforms, allowing eLearning professionals to identify areas for improvement. Moreover, it can help create personalized learning experiences that cater to distinct learning needs and preferences. Finally, by regularly keeping track of data, data analysis can detect trends and make predictions that will guide your decision-making process for the future. All in all, utilizing data for eLearning contributes to the development of learning programs that adapt to the constantly changing needs of the digital world.
Steps And Techniques Of Data Analysis You Need To Know About
Data analysis is a process that can grant us access to valuable information as long as it is implemented correctly. Let’s take a look at the individual steps you need to take.
1. Establishing A Purpose
The first step of the process is also probably the most important. It’s the moment to reflect on your business problem or question and decide what types of eLearning analytics you are looking to collect and what you want to achieve through their analysis. For example, you may want to find the most ineffective modules in your online training program so that you can modify or replace them.
2. Data Collection
Guided by the observations and decisions made during the first step, you are ready to start collecting the necessary data. Usually, quantitative as well as qualitative data are needed, which can be accumulated through surveys, interviews, direct observation, etc. Make sure to find a depository or trusted cloud space where you can store and organize this information, as the volume is expected to be rather large.
3. Data Cleaning
Given the large amount of data available, it only makes sense that not all of it will be useful. Therefore, during this stage, you must sort through data and clean them up by deleting duplicates or outliers that don’t contribute to the final results. Although this process can be long and grueling for data analysts, it is necessary if you want the subsequent analysis process to be successful.
4. Data Analysis
At this stage, your data is clean, organized, and ready to be analyzed. There are various techniques or methods you can use to detect patterns and connections, through which you can find answers to your business question or make predictions for the future. Here are some of the most common data analysis techniques:
- Descriptive analysis involves the description of the main features of the dataset. For this reason, it utilizes measures such as mean, median, mode, etc. It’s a great technique for the identification of patterns and trends.
- Diagnostic analysis examines the available data with the purpose of discovering the cause-and-effect relations among them. It utilizes methods such as drill down, data mining, and correlations, among others.
- Predictive analysis is used to predict trends, correlations, and outcomes further down the road. It is a very common and popular analysis technique, as businesses want to be prepared for the future. It is usually based on statistical modeling and Machine Learning algorithms, to name a couple.
- Prescriptive analysis takes predictive analysis one step further by not just making predictions but also identifying the actions you need to take in the future. It’s a great method to use to optimize resource allocation and make data-based decisions.
- Inferential analysis is used to draw conclusions or make predictions about a larger population. It utilizes hypothesis testing, confidence intervals, and regression analysis. One could say that it combines insights from the previous analysis methods to reach generalized conclusions based on a sample population.
5. Data Visualization
The final step in the data analysis process is visualization. In other words, this is the stage where you put your findings into graphs, charts, or bullet points to present to others. Visualizing data analysis findings is especially important in eLearning since you often have to prove to—often, non-technical—stakeholders that your online program is producing the desired outcomes.
7 eLearning Analytics To Focus On For Optimal Results
Knowing the steps of the data analysis process is vital to reaching the right conclusions about the success of your eLearning project. But what also carries great importance is knowing which data you need to collect from your audience. Here is a brief overview of 7 common analytics that will help you determine the effectiveness of your online learning program.
User Satisfaction Rates
Online learner satisfaction is a very important analytic for organizations that want to develop successful eLearning programs. Using polls and surveys, you can gauge whether online learners are enjoying the learning experience you have created for them and even pinpoint the formats and modules they prefer the most.
Skill Acquisition
What you’re trying to achieve through eLearning is to help learners acquire new skills, not just complete as many modules and sessions as possible. Therefore, it’s important to monitor their level of proficiency throughout the learning process. eLearning analytics will paint a clear picture of how well learners are absorbing new information and whether they are able to implement it in a spot check.
Time Spent In Sessions
The amount of time learners spend in sessions can provide you with many valuable insights into the effectiveness of the learning program. For example, you can compare the estimated length of a session with the time learners actually spend on it. If completion requires more time than you planned, it could indicate an array of issues, such as missing information or a design flaw that causes delays.
Device Type
It’s important to know which devices your audience uses to attend your online learning course. What if the majority is using their smartphones but you have designed the course keeping in mind mostly devices with bigger screens, such as computers and tablets? What steps could you take to make eLearning more user-friendly for learners on smaller devices?
Easy Vs. Tricky Questions
Both easy and hard questions have their place in a learning course. The former is there to keep learners interested, and the latter is to challenge them. However, are the questions that almost all users get right on the first try supposed to be this easy, or do you need to change them? Similarly, are there learners struggling too much with tricky questions? Is there any way to nudge them forward when they get stuck?
Drop-Off Rates
This analytic refers to instances when users leave a page before completing what they intended to do. This could indicate a particularly hard module for which they don’t have the necessary information. It could also point to design mistakes, bugs, or glitches in the system. Pinpoint the problematic pages and figure out the root of the problem.
Learner Progress
Set milestones and deadlines along the course of the learning program during which you check learner progress. How far has the learner progressed after a week or a month? Learners who are significantly further ahead might benefit from more challenging material, while those who are behind may need easier content or external support to reach their goals.
Conclusion
Data analysis has become an indispensable part of the eLearning industry, introducing benefits many of us would have considered impossible before. Although they don’t need to become data analysts, all eLearning professionals need to be acquainted with the steps of the data analysis process in order to make the most of it. It’s also crucial to know which eLearning analytics to focus on, depending on the questions you want to answer. We hope the information we shared in this article has helped you understand more about the process of data analysis and how you can utilize it to accomplish your goals.
Source link